- Home
- Search
- Jonathan C Kao
- All Reviews
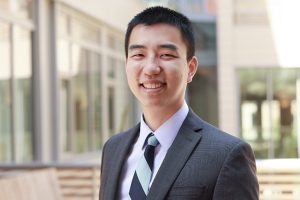
Jonathan Kao
AD
Based on 44 Users
This is probably the best introductory class to neural networks I have attended. It is very well structured and everything is built up in a purposeful way. It goes into the mathematical calculations behind deep learning, where the homework is about deriving and implementing the backpropagation algorithm for different neural network layers and functions. There are many opportunities to ask Prof Kao and the TAs questions during lectures and discussions, which they usually answer in meaningful ways.
On the more mixed side, the lectures in class are long (2 x 2 hours per week, not including discussions) and in this quarter, fell a bit behind schedule. As a result, many assignment deadlines and other topics had to be moved around, which could sometimes be confusing. Also worth mentioning is the workload, which is very high. A lot of derivations in the homework will require taking derivatives with respect to matrices, which can lead to tensor-sized derivatives easily. Coding the derivations in a performance-friendly way is also not trivial. As such, there is no shame in asking for help from students and teaching staff and it is generally encouraged, so you do not spend many nights figuring it out alone.
While the material is challenging it is communicated well and I personally feel I have a much better of what is happening when using neural networks from frameworks such as TensorFlow or PyTorch.
I really enjoyed this class with Kao. The concepts are really interesting, and he does a great job of explaining intuition and applications (including to his own research). He is very thorough and doesn't go too fast, and treats the subject with about as much mathematical rigor as you can without being in an actual math class.
He created slides in advance, then annotated them in class and posted the annotated PDFs for us to download. I never had to refer to the textbook to understand the material.
Kao is also very accommodating to students. He will move office hours, make corrections to materials, email quickly, and is always happy to look up the answer to a question if he does not know the answer already.
Kao a is an absolutely fantastic professor. His lectures are clear and engaging, and manage to break difficult concepts down into understandable chunks. He provides excellent slides, both annotated from class and unannotated originals, which are wonderful for studying. His slides often mention cutting-edge research in deep learning. Seriously, this is what a proper college class should feel like.
Although the class has listed prerequisites, they're not enforced. ECE 133A isn't really required (I didn't take it and did just fine). ECE/CS M146 isn't really necessary either, it's just background information that's mentioned in passing during lectures (I also hadn't taken it). You really do need to take a probability class though, even if it's not ECE 131A (STATS 100A or MATH 170E, etc. will do fine) or you'll be lost in the first half of the class.
The homeworks are quite time consuming, but there were only 5. They're a mixture of written math solutions and Python coding in Jupyter notebooks. It's helpful to have some exposure to Python before the class (even better if you already have familiarity with NumPy). The homeworks are pretty well spaced out, so there's plenty of time to complete them, and the TAs provide exceptional help during discussions (seriously, don't skip discussions. The TAs practically solve homework problems sometimes). Kao gives three "late days" across all the homework, so the deadlines are a little flexible.
Instead of a final, there is a final group project where you have to apply everything you learned in the quarter to a deep learning project. Kao provides a default project (in case you aren't creative, like me). It requires a fair amount of work, but it's due before finals week, so if you start early enough it doesn't interfere with studying for other classes. Getting a good group is essential.
Overall, this was one of the best courses I've taken at UCLA, and Kao is one of the best professors in the ECE department. If you're at all interested in machine learning, I highly recommend you take this class before you graduate. CS majors can probably petition it to count as an elective.
This class with Kao, I would rank as one of the top I've taken at UCLA. This was the first time he taught signals and systems, but he came into class very well prepared every single day. He really cared about making sure everyone understands the material best they can by giving a lot of intuition to the things he's teaching, and he mentioned how when he was an undergrad he did badly on the first midterm due to a lack of intuitive understanding. He also tends to mention how he uses the tools in 102 in his own work in neural prostheses, which is actually super cool to hear about.
There was a small pacing problem near the beginning of the class, because he would answer everyone's question in detail even though that's literally the next slide, but he later corrected that issue. Homework was challenging but doable – which to be honest is what should be the case. The grading curve was very lenient and he is committed to give everyone as high a grade as possible.
Basically, Kao is the best professor to take 102 with.
Kao is the first true 5/5 professor I've had at UCLA. While the material he taught in class was difficult, he truly does everything he can help students understand the material and do well on homework and exams. He also gives opportunities to improve your grade should you show improvement over the course of the class. He's just truly one of the most caring and understanding professors at this school, not to mention an overall nice guy and a good lecturer.
This is probably the best introductory class to neural networks I have attended. It is very well structured and everything is built up in a purposeful way. It goes into the mathematical calculations behind deep learning, where the homework is about deriving and implementing the backpropagation algorithm for different neural network layers and functions. There are many opportunities to ask Prof Kao and the TAs questions during lectures and discussions, which they usually answer in meaningful ways.
On the more mixed side, the lectures in class are long (2 x 2 hours per week, not including discussions) and in this quarter, fell a bit behind schedule. As a result, many assignment deadlines and other topics had to be moved around, which could sometimes be confusing. Also worth mentioning is the workload, which is very high. A lot of derivations in the homework will require taking derivatives with respect to matrices, which can lead to tensor-sized derivatives easily. Coding the derivations in a performance-friendly way is also not trivial. As such, there is no shame in asking for help from students and teaching staff and it is generally encouraged, so you do not spend many nights figuring it out alone.
While the material is challenging it is communicated well and I personally feel I have a much better of what is happening when using neural networks from frameworks such as TensorFlow or PyTorch.
I really enjoyed this class with Kao. The concepts are really interesting, and he does a great job of explaining intuition and applications (including to his own research). He is very thorough and doesn't go too fast, and treats the subject with about as much mathematical rigor as you can without being in an actual math class.
He created slides in advance, then annotated them in class and posted the annotated PDFs for us to download. I never had to refer to the textbook to understand the material.
Kao is also very accommodating to students. He will move office hours, make corrections to materials, email quickly, and is always happy to look up the answer to a question if he does not know the answer already.
Kao a is an absolutely fantastic professor. His lectures are clear and engaging, and manage to break difficult concepts down into understandable chunks. He provides excellent slides, both annotated from class and unannotated originals, which are wonderful for studying. His slides often mention cutting-edge research in deep learning. Seriously, this is what a proper college class should feel like.
Although the class has listed prerequisites, they're not enforced. ECE 133A isn't really required (I didn't take it and did just fine). ECE/CS M146 isn't really necessary either, it's just background information that's mentioned in passing during lectures (I also hadn't taken it). You really do need to take a probability class though, even if it's not ECE 131A (STATS 100A or MATH 170E, etc. will do fine) or you'll be lost in the first half of the class.
The homeworks are quite time consuming, but there were only 5. They're a mixture of written math solutions and Python coding in Jupyter notebooks. It's helpful to have some exposure to Python before the class (even better if you already have familiarity with NumPy). The homeworks are pretty well spaced out, so there's plenty of time to complete them, and the TAs provide exceptional help during discussions (seriously, don't skip discussions. The TAs practically solve homework problems sometimes). Kao gives three "late days" across all the homework, so the deadlines are a little flexible.
Instead of a final, there is a final group project where you have to apply everything you learned in the quarter to a deep learning project. Kao provides a default project (in case you aren't creative, like me). It requires a fair amount of work, but it's due before finals week, so if you start early enough it doesn't interfere with studying for other classes. Getting a good group is essential.
Overall, this was one of the best courses I've taken at UCLA, and Kao is one of the best professors in the ECE department. If you're at all interested in machine learning, I highly recommend you take this class before you graduate. CS majors can probably petition it to count as an elective.
This class with Kao, I would rank as one of the top I've taken at UCLA. This was the first time he taught signals and systems, but he came into class very well prepared every single day. He really cared about making sure everyone understands the material best they can by giving a lot of intuition to the things he's teaching, and he mentioned how when he was an undergrad he did badly on the first midterm due to a lack of intuitive understanding. He also tends to mention how he uses the tools in 102 in his own work in neural prostheses, which is actually super cool to hear about.
There was a small pacing problem near the beginning of the class, because he would answer everyone's question in detail even though that's literally the next slide, but he later corrected that issue. Homework was challenging but doable – which to be honest is what should be the case. The grading curve was very lenient and he is committed to give everyone as high a grade as possible.
Basically, Kao is the best professor to take 102 with.
Kao is the first true 5/5 professor I've had at UCLA. While the material he taught in class was difficult, he truly does everything he can help students understand the material and do well on homework and exams. He also gives opportunities to improve your grade should you show improvement over the course of the class. He's just truly one of the most caring and understanding professors at this school, not to mention an overall nice guy and a good lecturer.