- Home
- Search
- Sriram Sankararaman
- All Reviews
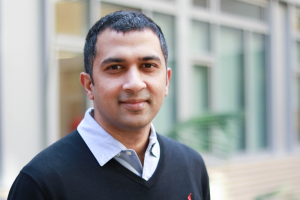
Sriram Sankararaman
AD
Based on 16 Users
grades were curved down. homework 50%, midterm 20%, and final 30%. if ur exam scores on midterm and final are higher than the median, and did all the homework, u should secure a B+ at least. not a easy class if u have no prior knowledge in ML.
Professor Sankararaman is an amazing lecturer, and he explains concepts in a clear and concise manner. For my quarter, there were eight quizzes in total (pretty much one per week), which was somewhat inconvenient. However, I learned a lot from this class, and it was overall a highly enjoyable experience. I would definitely recommend taking this class with this professor.
Siriam is an awesome professor. The class is very well-organized. There are several TAs who each hold lots of office hours throughout the week. The only complaint I have is grades were curved down at the end of the quarter.
This is a great class to take. The concepts are covered very well and the tests and homework’s are very fair. The material gets harder after week 5 as you do kernels and SVMs, so make sure to keep attending lecture. The grading scheme is tough so make sure you don’t lose points on the homework
Take it. The professor is very passionate about teaching and give clear instructions on what is going on every lecture. The slides are not very creative but they are clear enough even for self-study. The homework and problem sets are fairly assigned and graded. This class does not involve a lot of coding, so it is one of the easiest CS upper I have ever taken. He will give skeleton code, and all you have to do is to fill in the "to do" parts according to instructions, where everything is done in python. For lectures and exams, I feel it is more math and stats focused, but with the foundation is Stats 100A or other equivalents, one will be fine on those stuff. Honestly, easy A for a CS upper.
Class structure: Problem sets every two weeks, quizzes every week, final at the end
Lectures: engaging prof and great slides mean lectures were (mostly) easy to listen to. I watched them a day after on 1.25 or 1.5 speed, and that was great for me. I downloaded all the slides to reference later on.
PSets: if you watched lectures, know how to do some advanced calculus, and have a copy of the slides handy, you should be able to do all the conceptual problems in these PSets (roughly 50% of the points on each PSet). Additionally, they encourage you to discuss with other classmates to "check answers" and whatnot. The coding portions at the end of the PSets (50%) are super fun and straightforward. They give very detailed instructions and you get to see how machine learning works on real datasets.
Quizzes: also very straightforward. Plugging numbers into formulas (have to know which formula to use!) and having the sides handy to ctrl+F is imperative. I found that there was more than enough time to answer each question. They are all multiple choice.
Final: basically a long quiz.
Nice prof, cool material, good format
This is an amazing class. I can't recommend Professor Sankararaman enough. He is able to distill complex ideas into easy-to-understand and interesting lectures. His slides are slick, clear, and thorough. He also posted lecture videos online our year. However, I highly recommend going to class because it is really easy to fall behind if you rely on just the videos. This class is quite difficult. If this is your first machine learning class, you will have to put in a significant amount of effort to truly understand the material and get an A. Before the class starts, I recommend going over your Math 32A and 33A notes. You should be comfortable with multivariable calculus and linear algebra. You should also have taken a proper probability and statistics course beforehand. The projects and homeworks are pretty interesting. You'll be exposed to many different ML models and techniques such as decision trees, linear/polynomial regression, SVMs, PCA, boosting, HMMs, and clustering.
An overview of machine learning topics. Every lecture is worth watching and the pacing is quite fast in lecture, so record things you don't understand to review and REALLY understand. Weekly quizzes aren't hard (remember to do them!), homeworks are every two-three weeks which consist of a few multi-part questions (mostly computations or small exercises using concepts learned), and then a coding section. The coding parts are quite well-designed and easy to adapt to. The final was a gradescope multiple choice exam. Bloody awesome :)
I learned far more from this class than from m148. M146 with Sriram was engaging, challenging, and interesting, without being a total chore on workload (unlike m148). He lectures fairly well, though as with all learning done at UCLA, you learn better reconciling his explanations in lecture with other resources online.
Overall, to succeed in his M146, attend/watch every lecture (they're pretty good! - and the textbook doesn't exactly follow lecture), DON'T forget about the quizzes (set reminders), and start the homeworks fairly early like a normal good student would. They aren't extremely time consuming, however.
Highly recommend this class for those wanting a better mathematical foundation in machine learning and knowledge of the basic algorithms. The homeworks are mostly math problems and proof related to machine learning concepts, and usually the last problem involves programming one of the algorithms you learned in class. The tests are pretty much the same, minus the programming parts.
Sriram was a fine professor. He could be a little eccentric and sometimes go too quickly with the concepts + math proofs, but for the most part he and his TAs did a good job of making sure you could understand the concepts and the homeworks. Overall I recommend him as a teacher.
grades were curved down. homework 50%, midterm 20%, and final 30%. if ur exam scores on midterm and final are higher than the median, and did all the homework, u should secure a B+ at least. not a easy class if u have no prior knowledge in ML.
Professor Sankararaman is an amazing lecturer, and he explains concepts in a clear and concise manner. For my quarter, there were eight quizzes in total (pretty much one per week), which was somewhat inconvenient. However, I learned a lot from this class, and it was overall a highly enjoyable experience. I would definitely recommend taking this class with this professor.
Siriam is an awesome professor. The class is very well-organized. There are several TAs who each hold lots of office hours throughout the week. The only complaint I have is grades were curved down at the end of the quarter.
This is a great class to take. The concepts are covered very well and the tests and homework’s are very fair. The material gets harder after week 5 as you do kernels and SVMs, so make sure to keep attending lecture. The grading scheme is tough so make sure you don’t lose points on the homework
Take it. The professor is very passionate about teaching and give clear instructions on what is going on every lecture. The slides are not very creative but they are clear enough even for self-study. The homework and problem sets are fairly assigned and graded. This class does not involve a lot of coding, so it is one of the easiest CS upper I have ever taken. He will give skeleton code, and all you have to do is to fill in the "to do" parts according to instructions, where everything is done in python. For lectures and exams, I feel it is more math and stats focused, but with the foundation is Stats 100A or other equivalents, one will be fine on those stuff. Honestly, easy A for a CS upper.
Class structure: Problem sets every two weeks, quizzes every week, final at the end
Lectures: engaging prof and great slides mean lectures were (mostly) easy to listen to. I watched them a day after on 1.25 or 1.5 speed, and that was great for me. I downloaded all the slides to reference later on.
PSets: if you watched lectures, know how to do some advanced calculus, and have a copy of the slides handy, you should be able to do all the conceptual problems in these PSets (roughly 50% of the points on each PSet). Additionally, they encourage you to discuss with other classmates to "check answers" and whatnot. The coding portions at the end of the PSets (50%) are super fun and straightforward. They give very detailed instructions and you get to see how machine learning works on real datasets.
Quizzes: also very straightforward. Plugging numbers into formulas (have to know which formula to use!) and having the sides handy to ctrl+F is imperative. I found that there was more than enough time to answer each question. They are all multiple choice.
Final: basically a long quiz.
Nice prof, cool material, good format
This is an amazing class. I can't recommend Professor Sankararaman enough. He is able to distill complex ideas into easy-to-understand and interesting lectures. His slides are slick, clear, and thorough. He also posted lecture videos online our year. However, I highly recommend going to class because it is really easy to fall behind if you rely on just the videos. This class is quite difficult. If this is your first machine learning class, you will have to put in a significant amount of effort to truly understand the material and get an A. Before the class starts, I recommend going over your Math 32A and 33A notes. You should be comfortable with multivariable calculus and linear algebra. You should also have taken a proper probability and statistics course beforehand. The projects and homeworks are pretty interesting. You'll be exposed to many different ML models and techniques such as decision trees, linear/polynomial regression, SVMs, PCA, boosting, HMMs, and clustering.
An overview of machine learning topics. Every lecture is worth watching and the pacing is quite fast in lecture, so record things you don't understand to review and REALLY understand. Weekly quizzes aren't hard (remember to do them!), homeworks are every two-three weeks which consist of a few multi-part questions (mostly computations or small exercises using concepts learned), and then a coding section. The coding parts are quite well-designed and easy to adapt to. The final was a gradescope multiple choice exam. Bloody awesome :)
I learned far more from this class than from m148. M146 with Sriram was engaging, challenging, and interesting, without being a total chore on workload (unlike m148). He lectures fairly well, though as with all learning done at UCLA, you learn better reconciling his explanations in lecture with other resources online.
Overall, to succeed in his M146, attend/watch every lecture (they're pretty good! - and the textbook doesn't exactly follow lecture), DON'T forget about the quizzes (set reminders), and start the homeworks fairly early like a normal good student would. They aren't extremely time consuming, however.
Highly recommend this class for those wanting a better mathematical foundation in machine learning and knowledge of the basic algorithms. The homeworks are mostly math problems and proof related to machine learning concepts, and usually the last problem involves programming one of the algorithms you learned in class. The tests are pretty much the same, minus the programming parts.
Sriram was a fine professor. He could be a little eccentric and sometimes go too quickly with the concepts + math proofs, but for the most part he and his TAs did a good job of making sure you could understand the concepts and the homeworks. Overall I recommend him as a teacher.